Inside The Life Sciences Industry's Use Of AI-Integrated High-Speed Cameras

By John Oncea, Editor
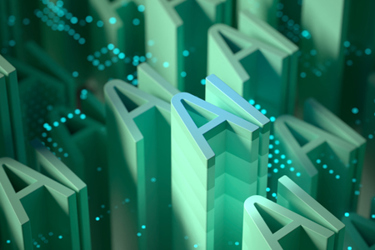
Integrating artificial intelligence into high-speed cameras enables real-time analysis, resulting in increased accuracy, improved planning, and better outcomes.
Loosely defined, the life sciences industry is a grouping of disciplines that center around the study of living organisms and life processes such as biology, medicine, and agriculture. As with many modern industries, life sciences is evolving and now requires more of a multi-discipline approach with biologists, chemists, and robotics and automation engineers working together to develop new solutions.
Among the technological transformations occurring in life sciences is the integration of high-speed cameras with artificial intelligence (AI), particularly in biomedicine and oncology research. This fusion enables real-time analysis of complex biological processes, offering unprecedented insights into disease mechanisms and potential therapeutic interventions. Over the past year, significant advancements have been made in this domain, underscoring its critical role in enhancing diagnostic accuracy and patient outcomes.
What Is Life Sciences?
The life sciences industry encompasses businesses and organizations focused on the research, development, and manufacturing of products and services related to living organisms, including pharmaceuticals, biotechnology, medical devices, and more, to improve human and animal health and well-being.
According to Fishers, IN – a city that has invested in attracting life sciences businesses and has 59% of its labor force working in the field – life sciences impact our lives and well-being every day. “From the medicines developed by pharmaceutical companies to cure, treat, and prevent disease, to the medical device companies that create and manufacture surgical tools, the life sciences industry is critical in improving individual and public health outcomes, in addition to the quality of life.”
The scope of the life sciences industry is broad and includes, but is not limited to:
- Pharmaceuticals: Development and production of drugs and medicines.
- Biotechnology: Utilizing biological systems and organisms to create products and technologies.
- Medical Devices: Designing, manufacturing, and developing medical equipment and tools.
- Biomedical Technologies: Technologies used in diagnostics, therapy, and research.
- Nutraceuticals and Cosmeceuticals: Products with nutritional and cosmetic benefits.
- Food Processing: Development of food products with health benefits.
- Environmental Sciences: Research and development related to environmental protection and sustainability.
The primary goal of the life sciences industry is to improve the quality of life for humans and animals through research, development, and the creation of innovative products and services. The life sciences industry plays a crucial role in advancing healthcare, biotechnology, and other related fields, contributing significantly to the economy and driving innovation.
Some of the more well-known life sciences companies are Merck, Pfizer, Novartis, Roche, Johnson & Johnson, AbbVie, GSK, Thermo Fisher Scientific, Altos Labs, TG Therapeutics, and KBI Biopharma.
These companies and others like them are focusing on digital transformation achieved by advancements in cloud computing, generative AI, and other digital technologies, writes Deloitte. “About 60% of executives cited gen AI or digital transformation as key emerging trends they are closely monitoring. Moreover, nearly 60% of executives plan to increase gen AI investments across the value chain, suggesting that companies are moving beyond initial pilot projects and are beginning to realize substantial value from adopting these technologies at scale.”
Deloitte opines this can be accomplished if industry leaders prioritize strategic issues that can most benefit from gen AI, and then clearly define and communicate these issues to stakeholders and technical experts. They also note the life sciences industry needs to create the necessary infrastructure to deploy gen AI models effectively at scale.
“In the future, this may evolve further,” Deloitte writes. “As Akiko Amakawa, corporate strategy officer and CEO chief of staff at Takeda, shared in an interview, ‘Currently, digital investments are driven by specific initiatives, but we will need to develop a prioritization framework to manage these investments as a portfolio just like we have been managing our R&D pipeline.
“The challenge lies in developing consistent metrics, as digital projects span diverse goals including risk management, operational efficiency, and customer satisfaction that don’t easily compare under one measure like ROI. Creating a meaningful prioritization framework for these investments is still evolving.’”
Advancements In AI-Integrated Imaging Systems
One notable development is the creation of AI-powered digital imaging systems designed to expedite cancer biopsy results. Researchers at the University of Waterloo introduced the Photon Absorption Remote Sensing (PARS) system, which significantly accelerates the cancer detection process, delivering diagnoses within minutes.
This system employs lasers to generate detailed data from tissue samples, which are then analyzed by AI to produce standard histopathology images for pathologists. This innovation not only reduces patient anxiety associated with prolonged waiting periods but also streamlines surgical interventions, ensuring thorough removal of cancerous tissues during initial procedures.
Similarly, the integration of AI into microscopy has led to the development of data-driven microscopes capable of real-time analysis. According to arXiv, these intelligent systems incorporate feedback loops between data acquisition and analysis, optimizing imaging parameters such as speed, resolution, and field-of-view. By leveraging machine learning, these microscopes can adapt to dynamic biological environments, facilitating high-throughput biomedical applications like drug discovery.
Real-Time Analysis In Oncology Diagnostics
The application of AI-integrated high-speed imaging has profoundly impacted oncology diagnostics. For instance, Politico writes the development of the Clinical Histopathology Imaging Evaluation Foundation (CHIEF) model by Harvard Medical School represents a significant leap in cancer diagnosis. Trained on over 15 million images, CHIEF can accurately detect multiple cancer types, assess treatments, and predict survival rates. Its ability to link tumor cell patterns to specific genomic aberrations enables the suggestion of effective treatments without the need for costly DNA sequencing, thereby enhancing diagnostic precision and patient care.
In lung cancer care, Imaging Technology News writes that AI-powered continuums have been unveiled to support early detection and management. These systems utilize AI solutions to identify, measure, manage, and monitor lung nodules, facilitating earlier identification and referral for imaging or treatment pathways. By addressing challenges such as low screening appointment uptake rates, these AI-driven tools aim to improve patient outcomes through timely interventions.
Enhancing Personalized Medicine Through AI And High-Speed Imaging
The synergy between high-speed imaging and AI also has propelled personalized medicine forward. Generative AI, for example, is revolutionizing oncological imaging by offering new avenues for cancer detection and diagnosis, according to Oncotarget. These AI models can generate realistic medical images, aiding in the identification of subtle patterns indicative of specific cancer types. This advancement not only enhances diagnostic accuracy but also paves the way for tailored treatment strategies based on individual patient profiles.
Furthermore, Massive Bio writes the integration of AI in clinical trial matching platforms has streamlined patient enrollment in oncology studies. Massive Bio’s unveiling of an AI-driven platform at ASCO 2024 exemplifies this trend, offering personalized insights for clinical trial accessibility and thereby accelerating the development of novel cancer therapies.
Challenges And Future Directions
Despite these advancements, challenges remain in the widespread adoption of AI-integrated high-speed imaging systems. Ensuring data privacy and security, particularly in federated learning networks, is paramount.
These networks enable collaborative AI training across multiple institutions without sharing sensitive patient data, thus preserving privacy while enhancing the robustness of AI models. The expansion of such networks to real-time learning systems holds promise for continuous improvement in diagnostic accuracy and patient care.
Moreover, the integration of AI into existing clinical workflows necessitates careful consideration of regulatory and ethical implications. Establishing standardized protocols for the validation and deployment of AI-driven imaging systems is crucial to ensure their safe and effective use in clinical settings.
The convergence of high-speed cameras and AI has ushered in a new era in biomedical and oncology research, characterized by real-time analysis and enhanced diagnostic capabilities. These advancements have the potential to significantly improve patient outcomes through early detection, personalized treatment strategies, and streamlined clinical workflows. As the field continues to evolve, ongoing research and collaboration will be essential to address existing challenges and fully realize the transformative potential of AI-integrated high-speed imaging in life sciences.