Exploiting Optics Opportunities In 3D Sensing — Scaling Challenges
By Sabbir Rangwala, Patience Consulting
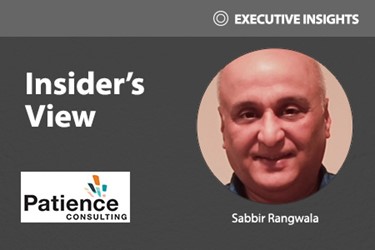
This article reviews key ideas discussed at the recent CLEO Conference — specifically, at the OIDA Executive Forum on the Exploding Role of Optics in Sensing — held May 14, 2018 in San Jose, Calif. It also includes my own thoughts and observations.
Keynote presentations from Microsoft’s Pat O’Connor and Loup Ventures’ Gene Munster provided excellent overviews of applications in optical sensing. Consumer applications include gaming, facial recognition in mobile phones, entertainment, and social networking. Augmented reality (AR) and virtual reality (VR) applications also were discussed. Further, business applications include training, education, and surgery through AR, as well as the emerging need for perception and movement automation for security, driverless cars, robotics, and trucking.
The presentations laid out the motivations for 3D optical sensing in terms of the tremendous application and revenue potential, as well as some of the challenges — like intelligent data fusion, computing and processing, compactness and, of course, cost.
The panel Mobility and Productivity – Seeing is Believing focused on the investment community — venture capital and investment banking. The panelists discussed the tremendous amounts of money being invested in 3D sensing — at this point, the number of companies doing LiDAR for driverless cars is significant (over 60, based on informal estimates). Securing new funding is becoming difficult and would occur only if the business plan truly offers a competitive advantage – in terms of approach, performance, team experience, deployability and cost. At this point, deployability, practical implementations and scalability are critical.
Discussion centered around the perception that optics is still a “cottage industry” and, unlike silicon micro-electronics industrialization, optics faces the challenge of integrating a wide variety of material systems. Progress in cost-reduction is being inhibited because wafer manufacturers are wary of following the silicon-like evolution of moving to larger wafer sizes pro-actively, rather than reactively. Panelists also discussed the need for, and imminent possibilities of, silicon photonics enabling low-cost, chip-scale solutions.
I agreed with most of the discussion, except for a few items. First, the obsession with moving to larger wafer sizes is misplaced. The more critical need is to dramatically increase quality, predictability, and yields of laser and detector devices from existing wafer sizes. This can be done by engineering more sophisticated metrology for in-process wafer fabrication processes to enable rapid process feedback, resulting in higher quality, lower scrap and, most importantly, improved predictability.
Developing well-understood process rules that device designers can use to ensure high Cpk designs also is critical, and can be structured robustly once a focus on improved performance in existing wafer sizes is embraced, and metrology and data become available to drive such rules. Additionally, the inability to certify that an optical chip will meet performance requirements at the wafer or chip level (KGD = Known Good Die) is a critical maturity issue.
Unless these shortcomings are addressed, moving to larger wafer sizes will only magnify the problem.
Another point to keep in mind is that the packaging costs are really what dominate optical component costs. The need for high opto-mechanical precision and stability in the assembly process, as well as significant levels of in-process and final unit testing, dominates the overall cost of a typical optical component.
Investment in faster and high-precision robotics is required to meet the scale and cost requirements for these new applications, as well as using KGD, so that testing can be reduced. Silicon photonics is attractive as a means for overcoming some of the challenges, but it is far away, and it is not clear that hybrid integration of active optics to silicon is a panacea for improving yields and costs, unless the challenges discussed above are overcome.
The panel Optical Networking: Perspectives from Asia featured an optical component manufacturer, Lumentum; an optical contract manufacturer, Fabrinet; and technology consulting firm, TechSearch International. An interesting comparison was made between needs for 3D sensing in consumer (cell phone) and business (automotive) applications. The key differences:
Application |
Product Life Cycles |
Tolerance to Failure |
Customer Base |
Reliability Requirements |
Consumer (Cell Phones) |
Short (1-2 years) |
Higher |
Narrow |
Modest |
Business (Automotive) |
Longer (3-5 years) |
Low |
Broad |
High |
Regarding consumer applications, the short product lifecycles for such products require optical companies to change designs rapidly, and to scale capacity in short timeframes. Further, optical companies typically work with one or two customers, supplying custom parts for short product life-cycles. Thus, losing a customer due to quality or cost issues can be devastating.
For business applications, the potential customer base is broader. One such application is driverless cars, for which 3D optical sensing provides a critical safety capability. Consequently, quality levels, design maturity, and reliability need to be at very high levels, since failure could cause loss of life. Reliable operation under harsh operating environments is a requirement for this application. So, the technical challenges are higher, the potential customer base broader, and product life-cycles are longer. However, performance and reliability requirements are more demanding.
Optical companies already are engaged in a significant way in the consumer applications of 3D sensing. The quantity of components shipped towards these applications over the past three years is comparable to volumes shipped for telecom, material processing and defense applications over the past 15-20 years. Rapid scaling of capacity is challenging, given the capital investments required and the allegiance to certain legacy processes associated with the “cottage industry” aspect of the optics industry.
Optical companies also are engaged in business applications like LiDAR for driverless cars, but the engagement is peripheral, rather than proactive. Apart from difficulties of pivoting from existing business, there are worries about whether regulatory, liability, and competitive issues in this space will delay deployment. Given the fragmented space in which automotive LiDAR operates today, it takes mindshare and resources to develop winning business and technical strategies as a component supplier. Additionally, there are concerns that the automotive space for suppliers is brutal in terms of cost and quality pressures, and whether it ultimately will be lucrative to the bottom line (lots of audience questions on whether 3D consumer sensing is profitable). But, optical companies should understand that the opportunity is huge, and they are the real enablers of this revolution — if they move forward aggressively, pivot successfully, and bring their significant IP and process knowledge to bear.
Another discussion in this panel focused on intelligent manufacturing — for example, applying machine learning to the significant amounts of data captured during the manufacturing operation to extract intelligence on eliminating certain testing steps. The point was made that, even today, every shipped laser is individually tested, requiring massive investments in space, people, equipment.
Artificial intelligence (AI), machine learning, and data mining can have a big impact on how optics manufacturing can scale beyond the “cottage industry” feel of the industry today. These techniques have been successfully implemented in various fields, including complex manufacturing industries.
Airbus, for example, created an AI system in which past experiences resolving assembly disruptions were captured in a knowledge-based system used to resolve assembly line problems and disruptions more efficiently.1 Problem patterns were mapped into similar prior patterns to identify and shortlist potential solution paths, without having to assemble the varied expertise typically required in brainstorming and troubleshooting meetings. This type of rapid troubleshooting could substantially help the optics industry to resolve manufacturing issues for high-volume consumer and business applications in 3D sensing.
Another big contributor to the relative immaturity of optics manufacturing today is the lack of predictability regarding product performance until later stages of manufacturing. The single biggest imperative is to provide predictability of the end product early in the process cycle — by implementing the kinds of capabilities discussed earlier, like metrology in wafer fabrication, and developing methods to assure KGD before the more expensive phases of packaging.
AI techniques excel at improving the predictability of complex systems when based on appropriately structured knowledge, logic, and learning algorithms.2 However, implementing such systems requires a comprehensive data strategy. The data needs to be selected and structured (in content, format, and relevance) with overall business imperatives in mind (high quality, predictability, yield, throughput, and cost). The investment is not trivial, but once made, provides an efficient path for the optics industry to effectively exploit the opportunities for 3D sensing in these massive emerging markets.
About The Author
Sabbir Rangwala founded Patience Consulting, LLC in November 2017. He specializes in perception, sensing, and machine learning for movement automation. Prior to this, he was President and Chief Operating Officer of Princeton Lightwave, where he successfully pivoted the company from a defense component supplier to a leading automotive LiDAR player, resulting in an acquisition by Argo.ai. Prior to this, Sabbir worked in various leadership roles in the optics arena for 25 years. He can be reached at srangwala@verizon.net
References:
- Sam Ransbotham et al., “Reshaping Business with Artificial Intelligence”, MIT Sloan Management Review, September 6, 2017.
- Ajay Agrawal, “The Economics of Artificial Intelligence”, McKinsey Quarterly, April 2018.